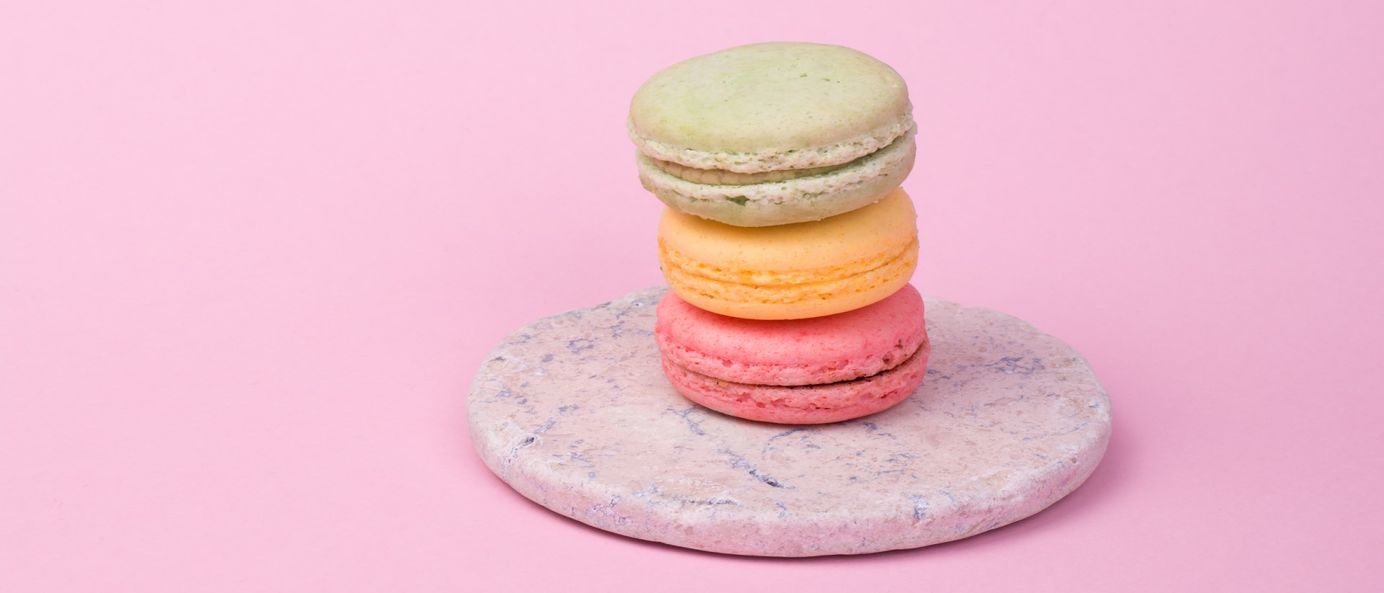
Elements of an Effective Data & Analytics Function
What does it take for an organisation to be able to perform analytics in a scalable (read: beyond Excel) manner? When I worked at Google, my instinctive answer would have been that you need analysts and a platform for them to work with. Easy. Yet working in the real world – outside the bubble of techno-privilege where data is generally organised, accessible, and reliable – made me realise there are a number of other elements you need. Yet before we dive in, we need to consider the type of team you are building.
Broadly speaking data and analytics teams come in two variants. There are those who only exist to govern the organisation's data, e.g. to make it easier to work with existing enterprise applications, and there are those who build and implement their own data products, whether data sets, tools, or models. You often find the former in financial institutions, where a Chief Data Officer might only have a handful of resources, with none of them necessarily technical. For this example we will consider the latter, with their three goals.
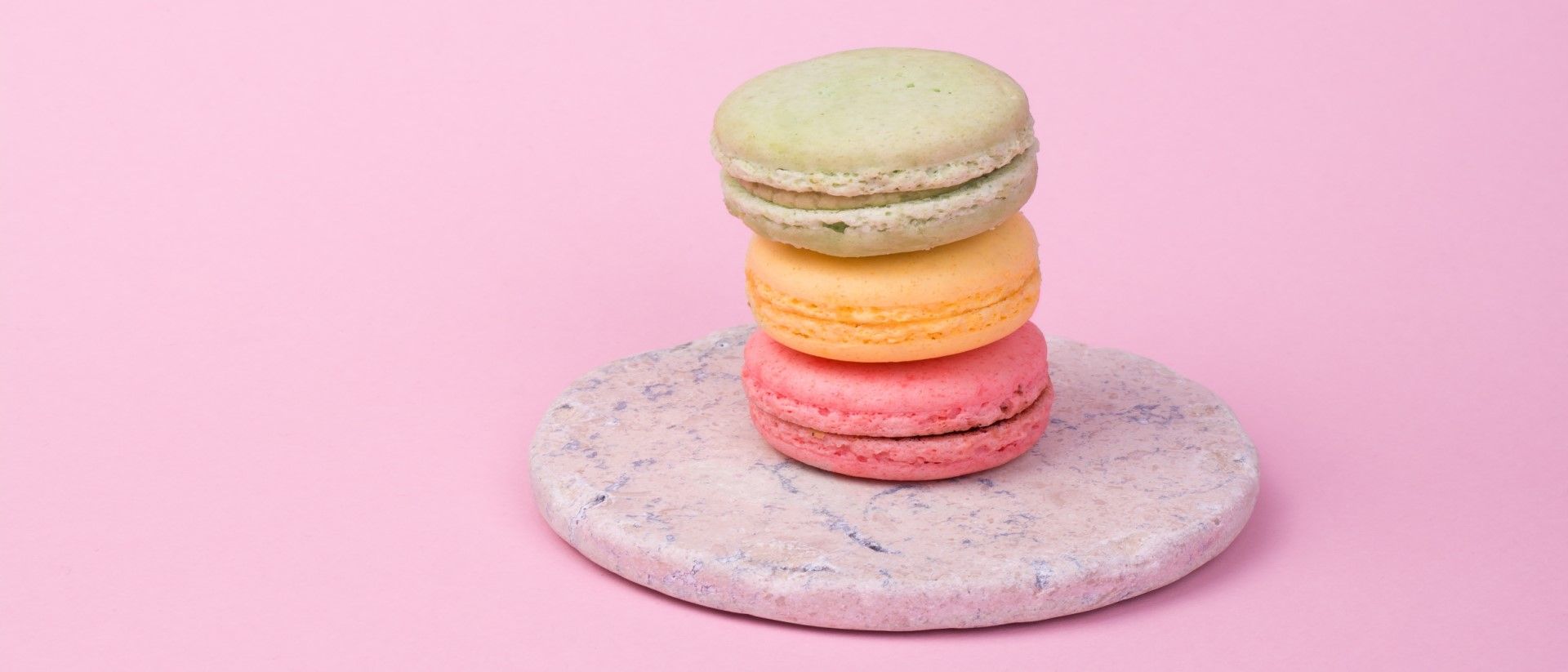
Firstly, they want to ensure that data is mapped and managed. This means having data architects who can not only bridge the business requirements and the underlying data, but are also able to structure the data in a way that makes sense. For example, how do you define customer data? Is it a first name, last name, email? Do you include social media handles? etc. You also need data quality and data governance resources, e.g. data stewards, who ensure that the data is trustworthy, and handled properly by the business.
Secondly, these teams need the ability to transform source data in ways that increases its value, either through improved usability, refining, or combining. They can also build models that are able to enrich data through classification and expansion, such as in customer segmentation and forecasting respectively. Doing this in a robust manner requires software engineering skills, found in roles such as data engineering and machine learning engineering. Ideally, you will also have data product managers to own the roadmap and ensure successful delivery. Why not project managers? More on that next week.
Assuming you manage your data platform, (cloud) devops engineering is an important function to have in your team. It will ensure that your engineers and analysts alike never need to worry about the data infrastructure or its performance. Security expertise is important too, but in most organisations your platform engineers will partner closely with the security architecture function under the CIO or CISO. This obviates the need to hire more scarce talent and can promote a holistic approach to cyber security.
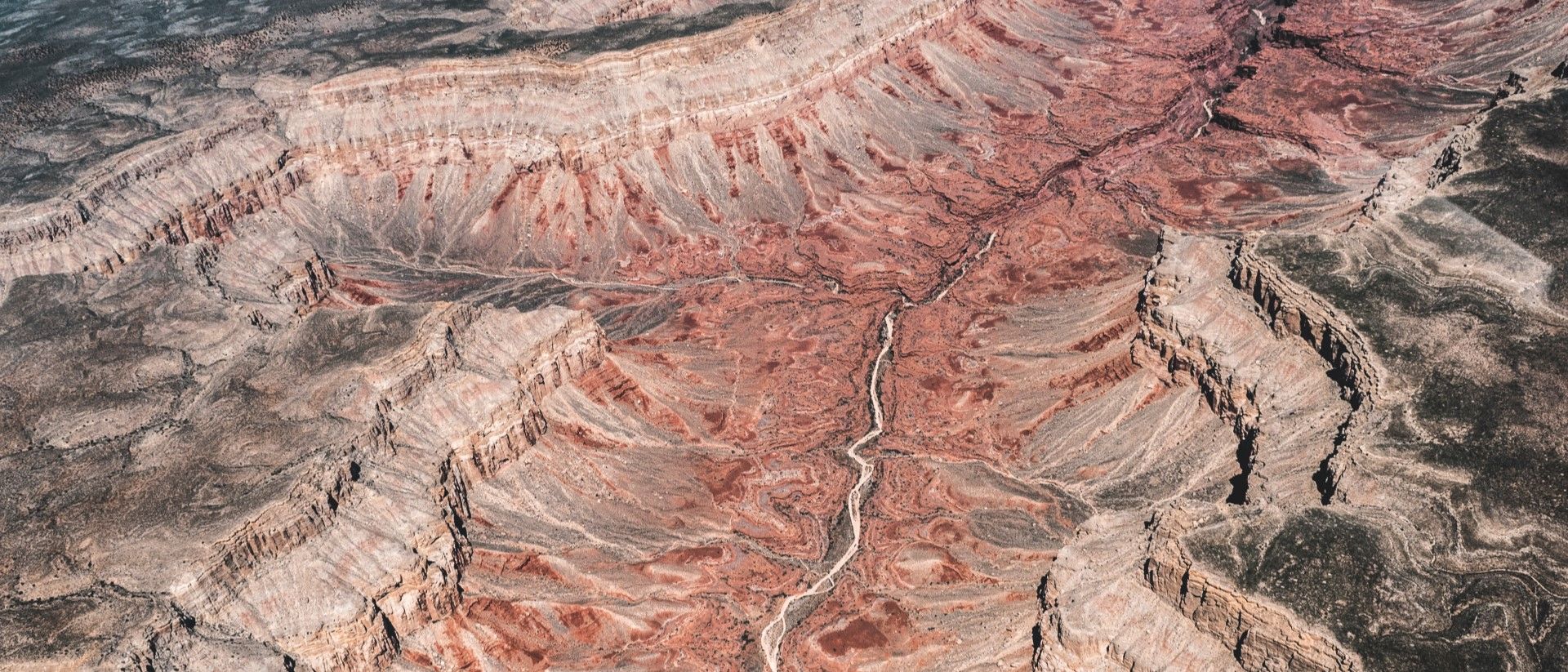
Thirdly, with reliable data and powerful data products in place, the team needs the skills to realise this value in the organisation. This can either be through a centralised function of data analysts – technical or non-technical – and data scientists, or through analytics community roles that develop and direct analytical talent embedded in teams across the organisation. Data ethics roles, even part time, are critical in organisations working with personal data or developing applications that have significant personal or societal impact.
Data architecture, data quality, data governance, data engineering, ML engineering, devops engineering, data product management, data analysts, data scientists, analytics community roles; clearly analysts cannot go it alone! I will caveat this by saying that some teams combine roles, e.g. having data engineers manage the platform, and that roles can be phased, e.g. few will need ML engineers when they get started, but the breadth of skills required to establish a modern data and analytics team poses a formidable challenge.
– Ryan
P.S. Do note that I have not mentioned any of the enabling roles required for this team to function. HR partners, finance managers, procurement specialists, lawyers, learning and development; these are all roles that are critical for a data and analytics team to function well in a large organisation. I guess the moral of the story is that no man is an island, particularly when it comes to data!
This week's topic was suggested by Andres Egoavil. Thank you!
Q* Newsletter
Join the newsletter to receive the latest updates in your inbox.