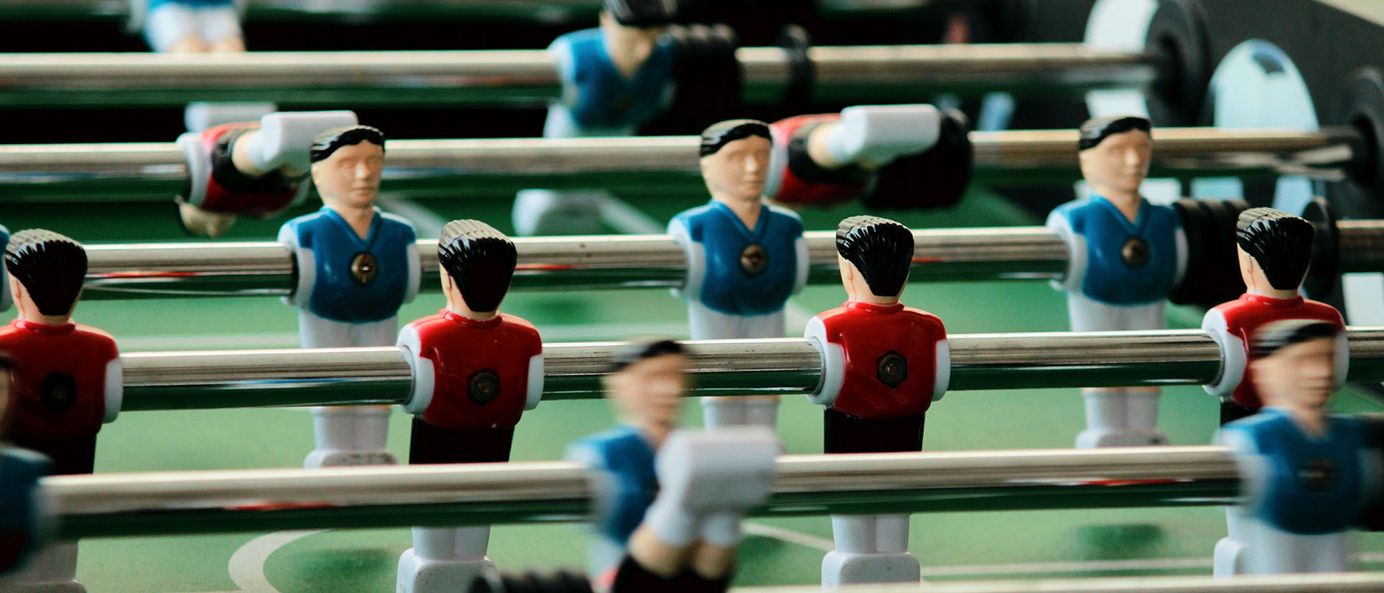
Embed Or Centralise? Where To Build Your Analytical Capabilities
When a business reaches the point when it decides it needs dedicated analyst or data science capabilities, one of the first questions that gets asked is ‘where should we put it’? There is no universal answer but there is another question that serves as a good starting point; should the capability be embedded in existing teams (with one or two analysts in mixed function teams), or should it be centralised into its own function (as dedicated teams of analysts working together with other functions)?
There are pros and cons of each approach and the right answer will depend on the existing organisational structure, and what and where the existing analytical capabilities are. Often, organic organisational growth will lead to a structure that is fragmented and inefficient. Taking the time to think through a deliberate strategy for structuring your analytical capabilities will pay off in the longer term as these capabilities grow in a structured way to support and adapt to the future needs of the business.
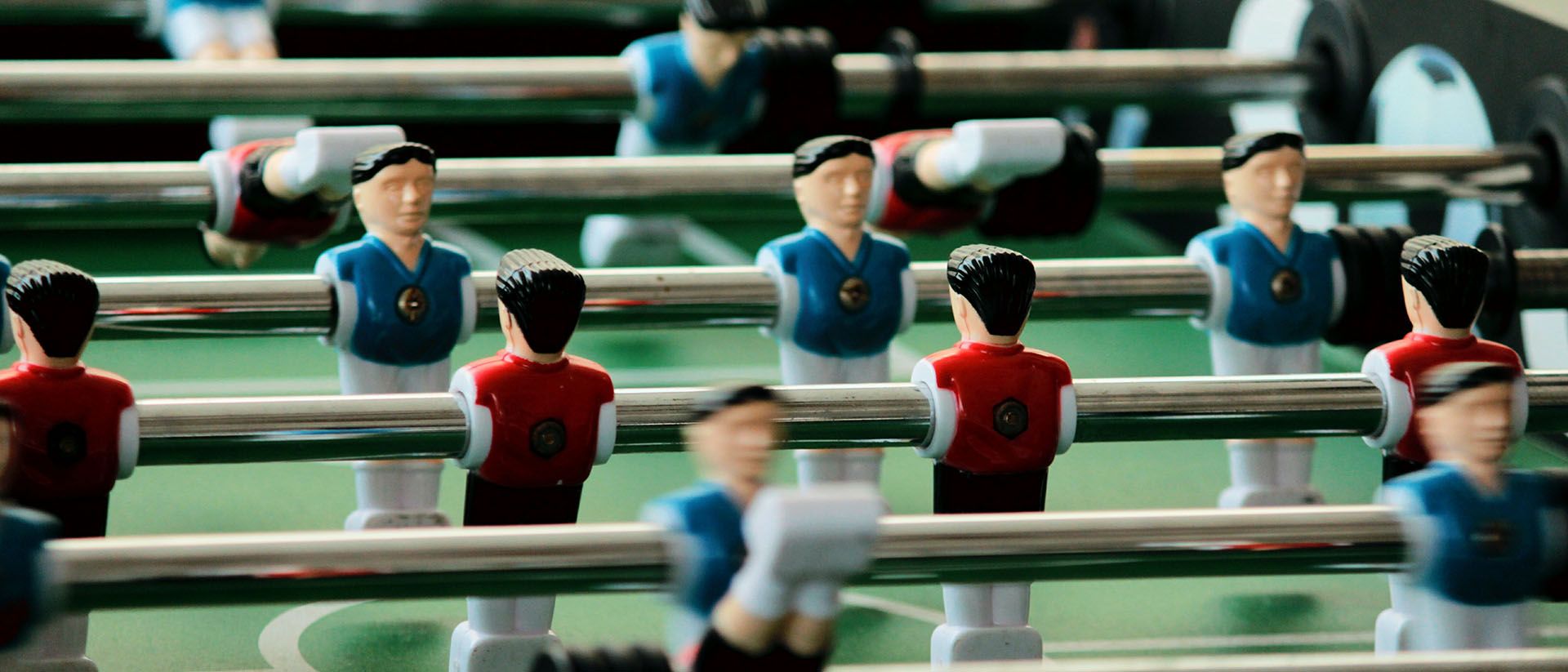
Let us start first with the ‘embedded analyst’ model. The key advantage to this model is that analysts are very close to the business units they support; they will likely gain deep domain expertise and really understand the business problems to be solved. This also brings benefits in hiring; the hiring manager can find the right person for the team from a fit and domain knowledge perspective. This model can be organisationally simpler, too; there is no need to create new structures or teams, so it is quicker to ‘get started’.
The embedded model has limitations, however. Analysts are siloed; without additional structures it is hard to share skills, techniques, code or knowledge. Having a non-analytical hiring manager trying to recruit someone with a specialist skill set they themselves do not have can be challenging. This problem does not stop after hiring either; it can be hard to support and grow analysts when they are isolated and fragmented across different teams. Organisationally, it is less scalable and efficient as the business grows; spare bandwidth goes to waste and work can not be distributed as easily as it would be across a team of analysts.
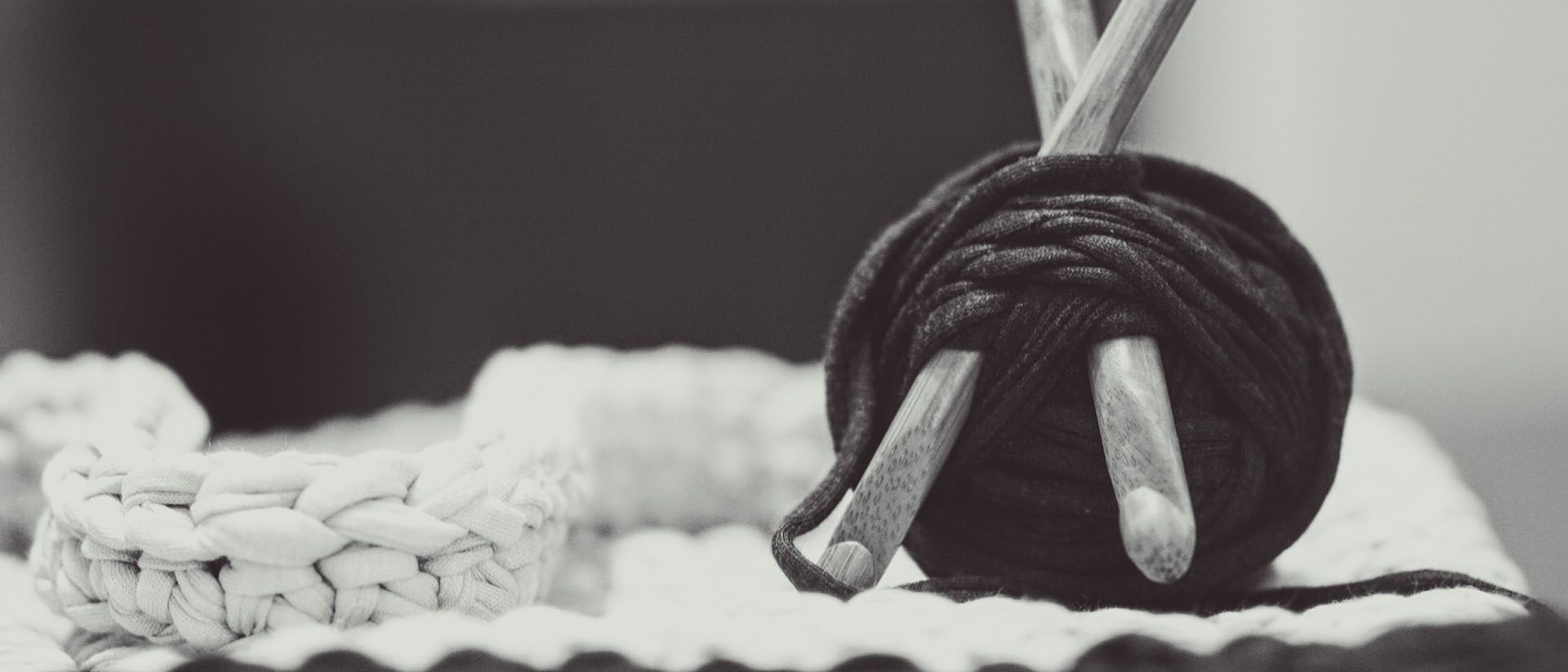
With the ‘centralised’ model, scalability is much easier; the team can be staffed as needed and capacity and bandwidth can be shared across partner/client teams. Knowledge, training and skills flow organically in the team and hiring the right skill set is easier although it is harder to build deep domain expertise. However, for many teams, building the required data science skills requires more investment than having sufficient domain expertise. Tension can sometimes occur between analyst/data science teams and the teams they work with, usually in terms of work prioritization and delivery. Good leadership can overcome this by building strong relationships and understanding between analytical and partner teams.
There may be a third way. Recently, modern organisational structures such as the helix have emerged in innovative businesses and these approaches may be well suited to data science and analytics functions. By separating out the development of the capability from the utilization of it, a 'best of both worlds' solution is obtained where a balance is struck between proximity to the business and specialisation of skills. For many organisations the cost of adopting such a model may be high and difficult to implement but for those willing to invest in change, the benefits are significant.
So what is the right model? It depends on the company; the size, the culture, the level of analytical maturity, the ability to attract and retain talent, and many other factors. In my opinion, for most organisations the flexible capacity and specialised leadership of the centralised model makes more sense. Even this specialised leadership alone brings enormous value with the right person in the role; a good data science leader can have influence and impact far beyond the sum of their teams. Just like any other organisational structure, however, there is no ‘one size fits all’ for data science. What are your thoughts on the issue? Please let us know on LinkedIn.
– Colm
Q* - Qstar.ai Newsletter
Join the newsletter to receive the latest updates in your inbox.