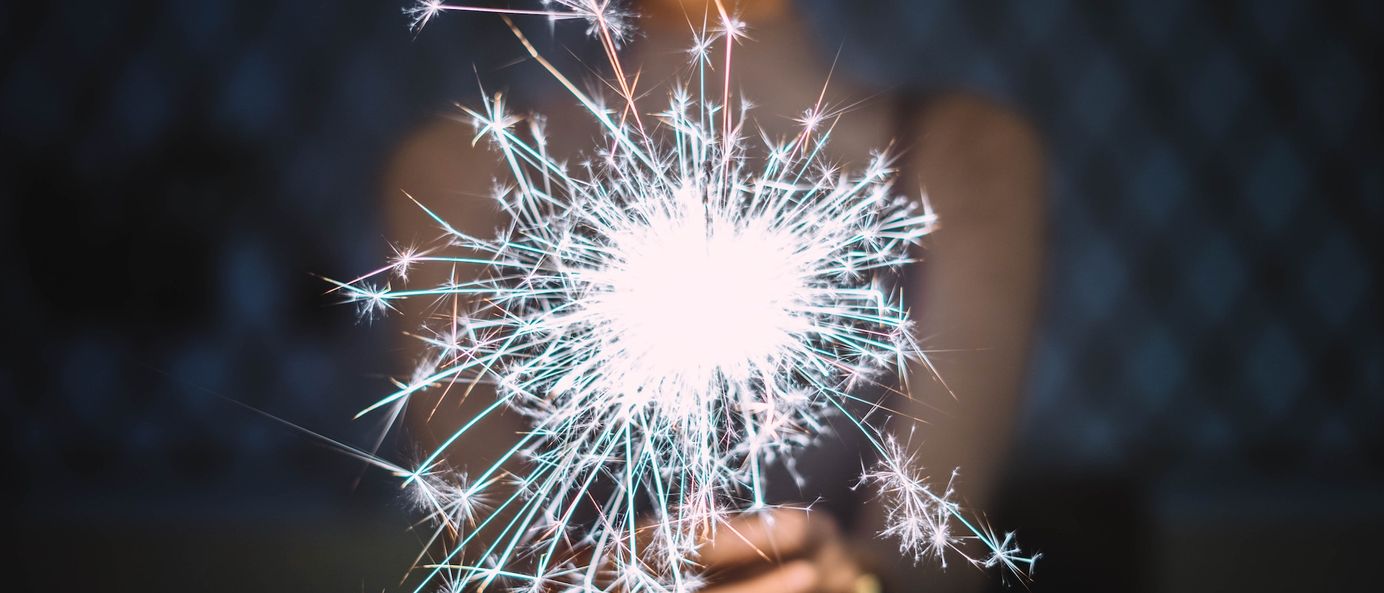
How Did Enterprise Data Science & AI Fare in 2019?
This is the time of year where many of us find ourselves reflecting on our performance. Did we stick with those New Year's resolutions, or not? Did we achieve what we wanted to achieve, or not? Similarly, let us consider how data science and AI fared in the enterprise over the past twelve months. This is a difficult topic to write about given that most organisations will sooner boast of successes than share their misgivings, so note that this is my personal view.
Let us start with the AI frontier, deep learning. While the leading machine learning conference, NeurIPS, was more popular than ever this year, not everyone was optimistic about the field's trajectory. Given the obsessions that exist with topping Kaggle leaderboards and questions of generalisation, the concerns raised are valid. Are we taking the right approach to solve the right problems? There is still a gulf between the public perception of AI and reality.
That said, even if we are heading into another AI winter, this will not make a difference to most companies. There are many who have not even reached the stage of implementing basic machine learning algorithms. Who cares about advanced techniques such as deep learning and reinforcement learning when the business is still trying to wrap their head around rudimentary classification or forecasting models. Most industries still have a long way to go on this front.
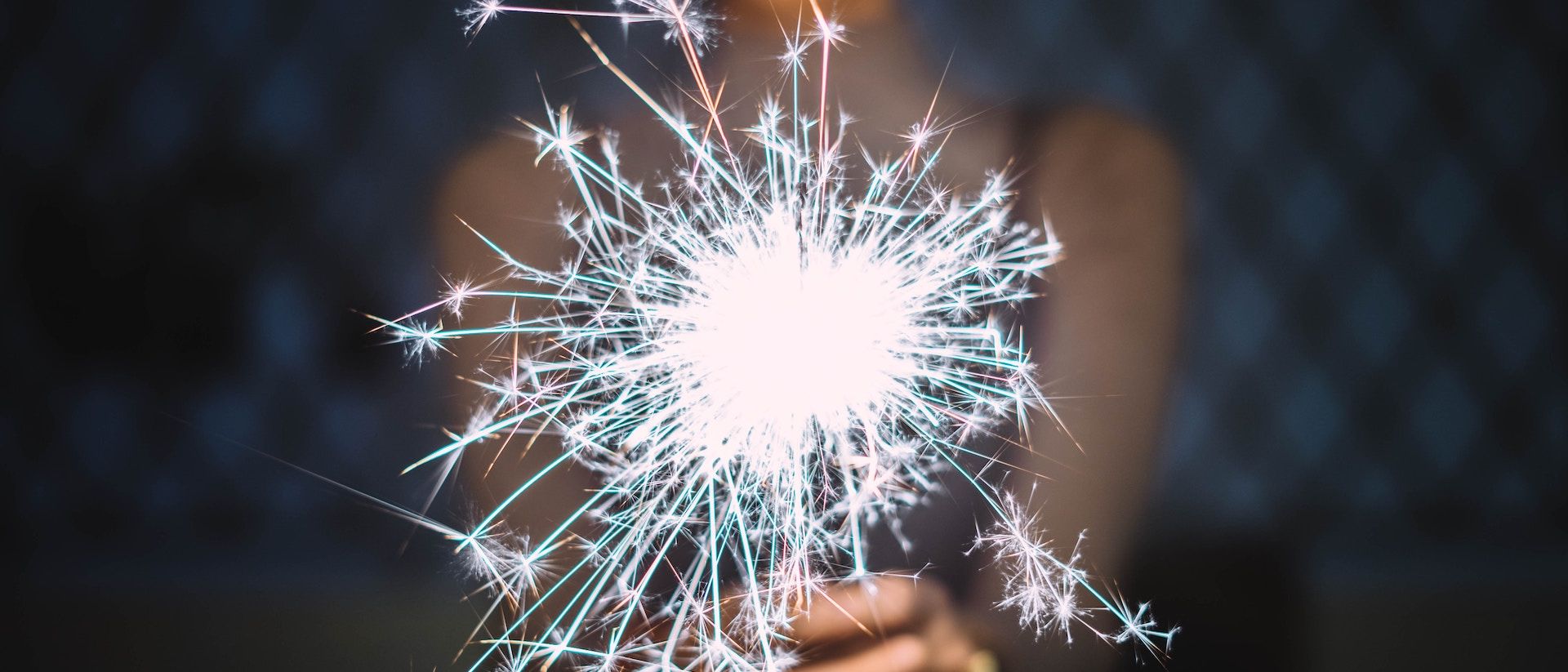
Though this might seem similar to last year’s outlook, there are now a number of significant differences. Firstly, the fundamental algorithms, tools, and libraries are becoming more comprehensive and more importantly much easier to use. The release of Tensorflow 2 was a major milestone. In tandem, we see that documentation and educational resources have improved markedly, with the machine learning engineer nanodegree I took a good example of this.
We are also seeing that big data platforms are becoming cloud data platforms. Hadoop is rapidly losing ground in many areas, with analytics teams opting for solutions that are more flexible, cost less, and are more performant. Even for those forced to run large on-prem clusters like banks or telcos, where will they ever find enough platform engineers? Increasingly these cloud migrations are not related to cost but driven by convenience, talent scarcity, and security.
It is interesting to note the development of managed enterprise AI platform, which make it easier to do feature engineering, model training, and model management. Many also offer built in safeguards like alerting for model drift which can help organisations prevent costly mistakes. Without a doubt there are companies wasting tens of millions on inaccurate machine learning deployments, they just do not realise it. Technology is now no longer the issue.
The first major challenge is talent, but I would argue not in the way you might think. The aforementioned resources are improving the quantity and quality of entry-level talent. Yet a lack of senior leadership is starting to hurt. To transform a large organisation means taking (informed) multi-million dollar risks, and being confident enough to convince the business to come on this journey. This requires deep domain expertise and there are few shortcuts.
Secondly, we need better solutions for data quality. Automated feature engineering is all well and good, but pointless without trusted data. To operate at scale organisations face a double challenge, both trying to collect vastly more data yet also increasingly having to work with non-technical domain experts in the business to guarantee its quality. As a McKinsey survey last year found, 92% of analytics initiatives fail to scale. We could do much better.
Thirdly, few organisations are investing enough in AI ethics. We need a mindset based not on "does this model work," but on "is this model fair?" Having experienced countless headcount reviews, my bet is that fewer than one in ten companies has the AI ethics or machine learning engineering talent they need to guarantee the fairness of their algorithms. This has nothing to do with malice and everything with the cost of talent and the complexity of the domain.
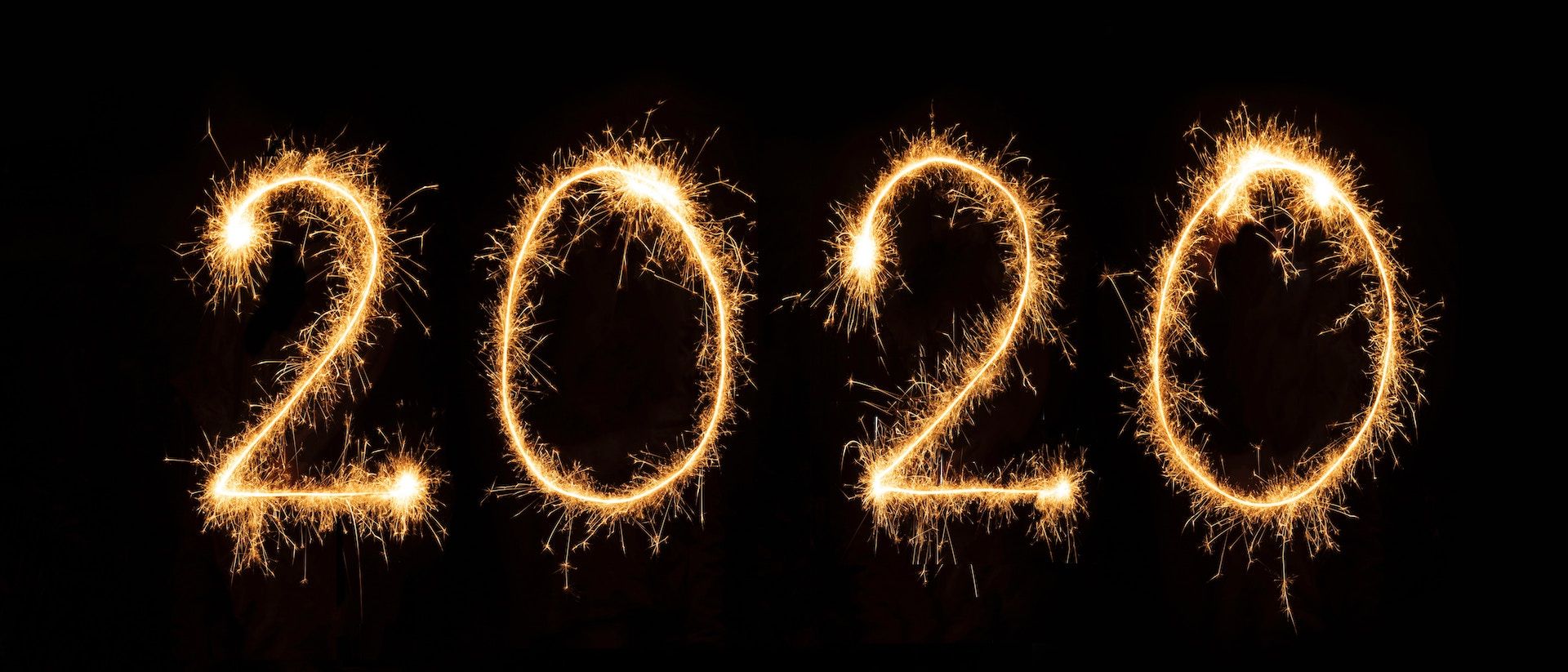
So where do we go in 2020? How do we prevent business leaders and rank and file alike from losing faith in the transformational role of analytics? While I feel there are multiple answers, let me posit the following. Because technology has gotten so much better, we need to abstract it. Forget citizen data scientists, as face it: most people do not grow up wanting to be scientists. Instead, how do we operationalise analytics in a way that makes it effortless for everyone?
What are the things people need to know in order to see analytics and AI as a part of their day-to-day lives? Educational efforts like Finland's Elements of AI are a good starting point. At the same time, we practitioners need to strike the right balance between building enthusiasm and remaining critical. When we think of using analytics, are we improving on the baseline, or are we just added a layer of technology? In the words of Cuba Gooding Jr: "Show me the money!"
Despite headwinds, 2020 will be a great year for data, analytics, and AI. Increasingly seen as a core business function alongside finance and marketing, data is feeling the growing pains and facing the scrutiny of maturity. Yet over the next decade, leaders and organisations who manage to navigate these challenges aptly will find themselves unlocking trillions of dollars in value and fundamentally improving the way that our societies operate. Happy New Year!
– Ryan
Q* - Qstar.ai Newsletter
Join the newsletter to receive the latest updates in your inbox.