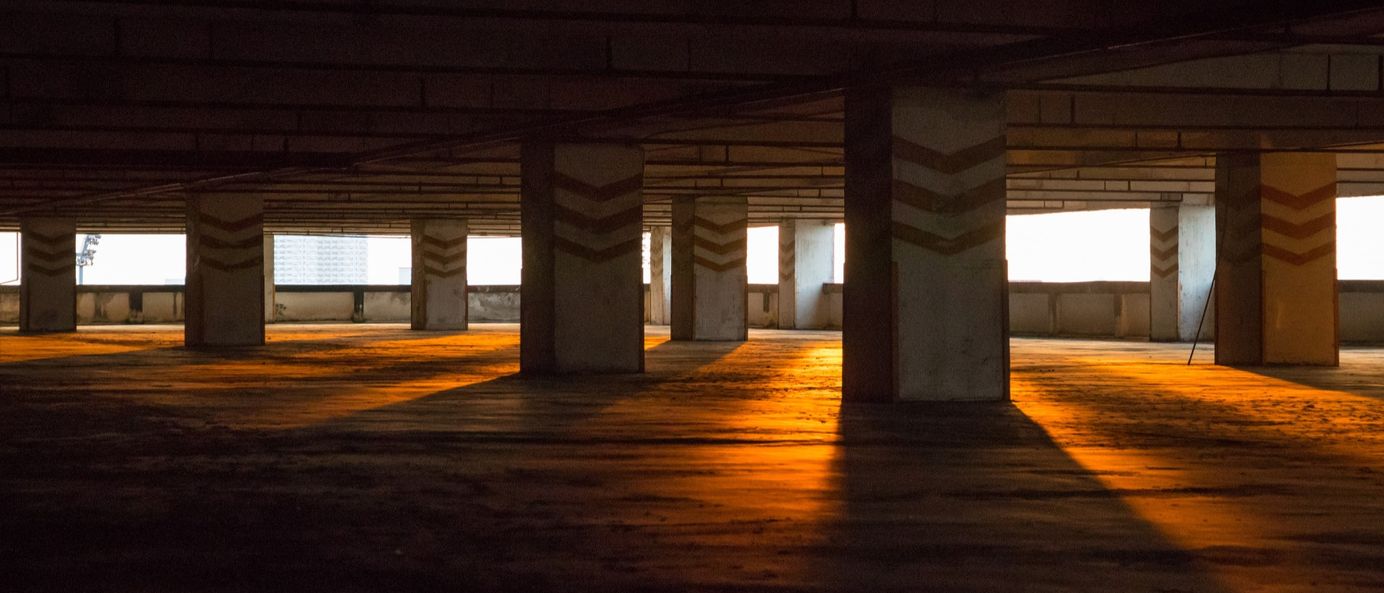
If You Are Serious About AI, You Had Better Get Serious About Data Quality
Ah, data quality. Almost as unpopular a cocktail party topic as data governance. Yet like that walking punchline Chandler Bing, data quality is about to enter its defining decade. It will most certainly have the last laugh. This is because you cannot build AI products that work right without the right data. In other words, investing in AI itself is useless without investing at least as much in data quality.
Why has data quality been easier to ignore in the past? Firstly, in many organisations only partial information has ever been used in analyses. Using partial data means that a few missing or erroneous data points do not make a major difference to the outcome of the analysis. This is particularly true if the analyses are a manual effort, whereby an analyst can tweak the data as they go along to correct any glaring errors. Missing a few records? Maybe you just hide them in your chart. Who needed them anyway?
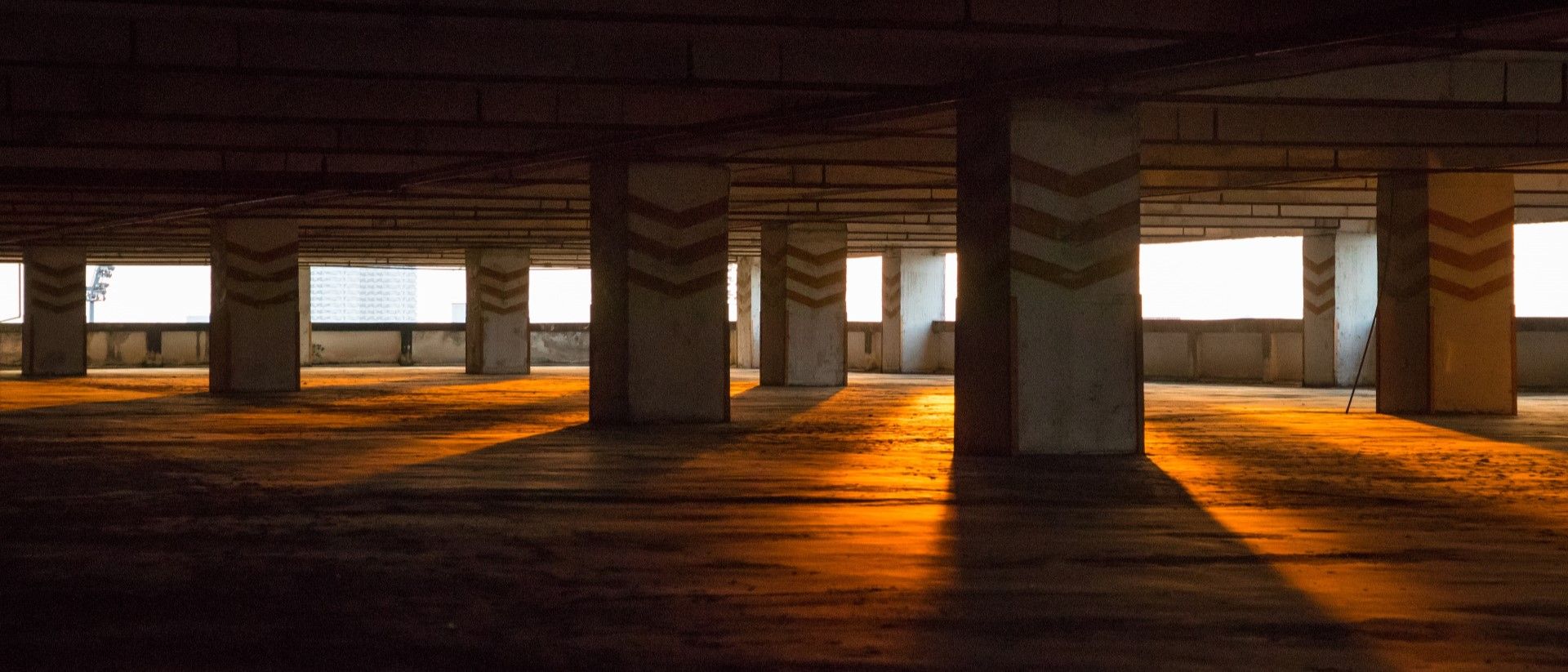
Secondly, in many organisations data has not truly been used to make decisions. Sure, in some cases it has informed decisions, but it is only in a minority of cases that you can draw a straight line between a data set and a real world outcome. In most organisation's analyses, there has always been a human interface between the data and the decision, in the form of an analyst or manager. Hell, in some cases that human would even fully disregard the data – but that is a topic for another week!
However, we now find ourselves in a world in which organisations are increasingly relying on analyses and algorithms that require both larger data sets and autonomy. For an example of the former, imagine how difficult it would be to build a recommendation engine with only a spreadsheet worth of past transactions. For an example of the latter, imagine deploying that engine on your website and having it check every suggestion with a human operator before presenting it to a customer.
This brings us to data quality. If we are feeding our machines more data and simultaneously setting them free to enact these decisions in the real world – in the case of autonomous vehicles, quite literally – then we need to guarantee the quality of the data. After all, machine learning means machines learning from examples. Whether your algorithm is a simple linear regression or a deep neural network, your performance will only ever be as good as the quality of the data that you feed it.
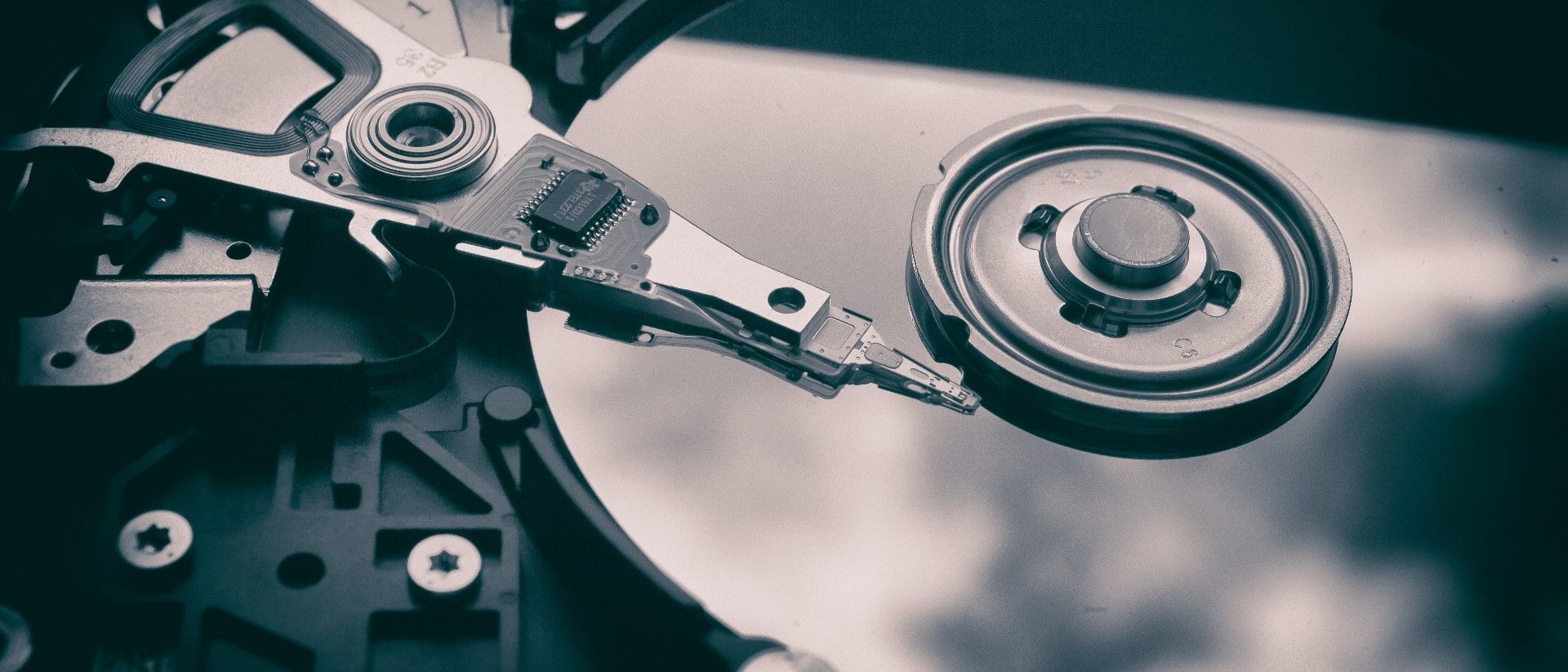
What does this mean for organisations? Primarily, we need to rehabilitate discussions around data quality. Just as people would feel embarrassed to present a board paper written in Comic Sans – and if they are not, they should – so we need people to feel bad when they make decisions based on shoddy data. No central function will ever be able to single-handedly address the data quality issues in an organisation. Instead, this needs to be a diffused team effort with everyone contributing.
Organisations should keep in mind that this is a marathon, not a sprint. Like seasoned athletes, they should grit their teeth and get on with improving data quality. Although crossing the finish line might not guarantee a successful AI strategy, it is a prerequisite. In another article we will see what this looks like in practice. Oh, and the fact that improved data quality also improves the quality of day-to-day decision making and reduces the organisations' risk profile? That is the icing on the cake.
– Ryan
Q* Newsletter
Join the newsletter to receive the latest updates in your inbox.