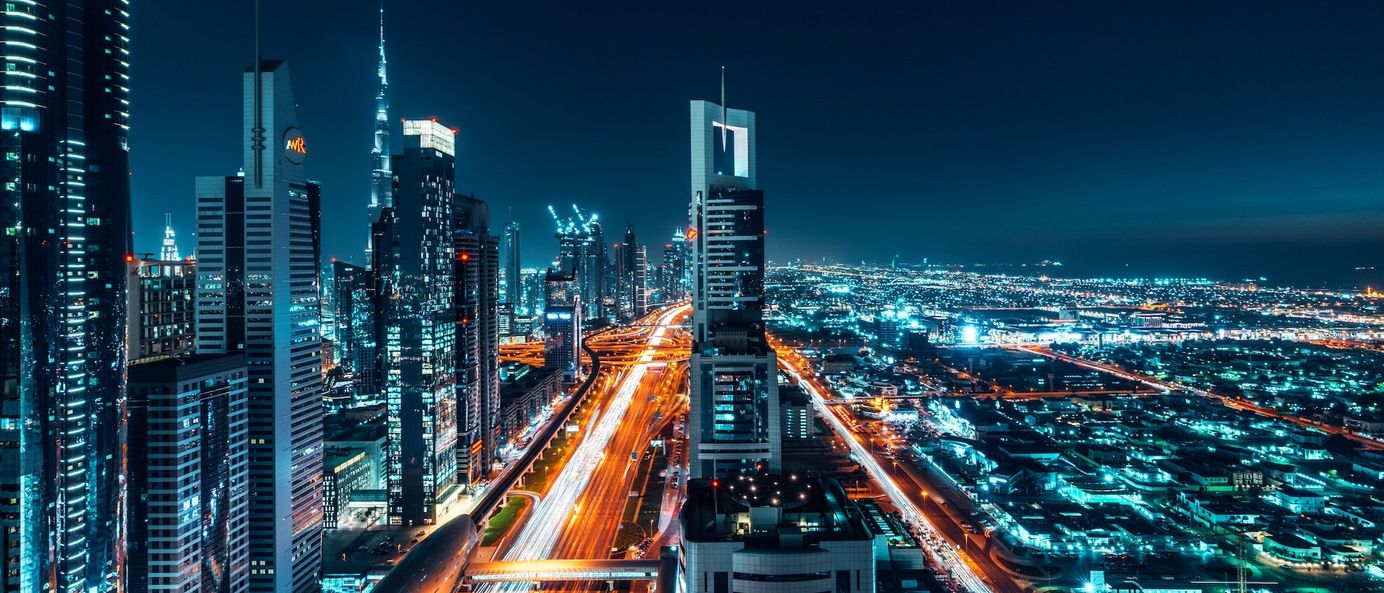
The Universal Language of Analytics
Arriving in a new country, you are faced with a lot of differences in customs, traditions, and culture. These changes are heightened when you move to a new region entirely. Even in a cosmopolitan city like Dubai, where English will get you far, you will miss a lot if you do not acquaint yourself with the fundamentals of Arab culture and history.
This type of change is not wholly dissimilar to entering a new industry. During my time at Google, I was fortunate to work with clients across sectors as diverse as entertainment, retail, finance, consumer goods, technology, and automotive. Even in sales and marketing, we had to constantly acquaint ourselves with new operating models, customs, and shibboleths, e.g. "moving the metal" to describe auto sales.
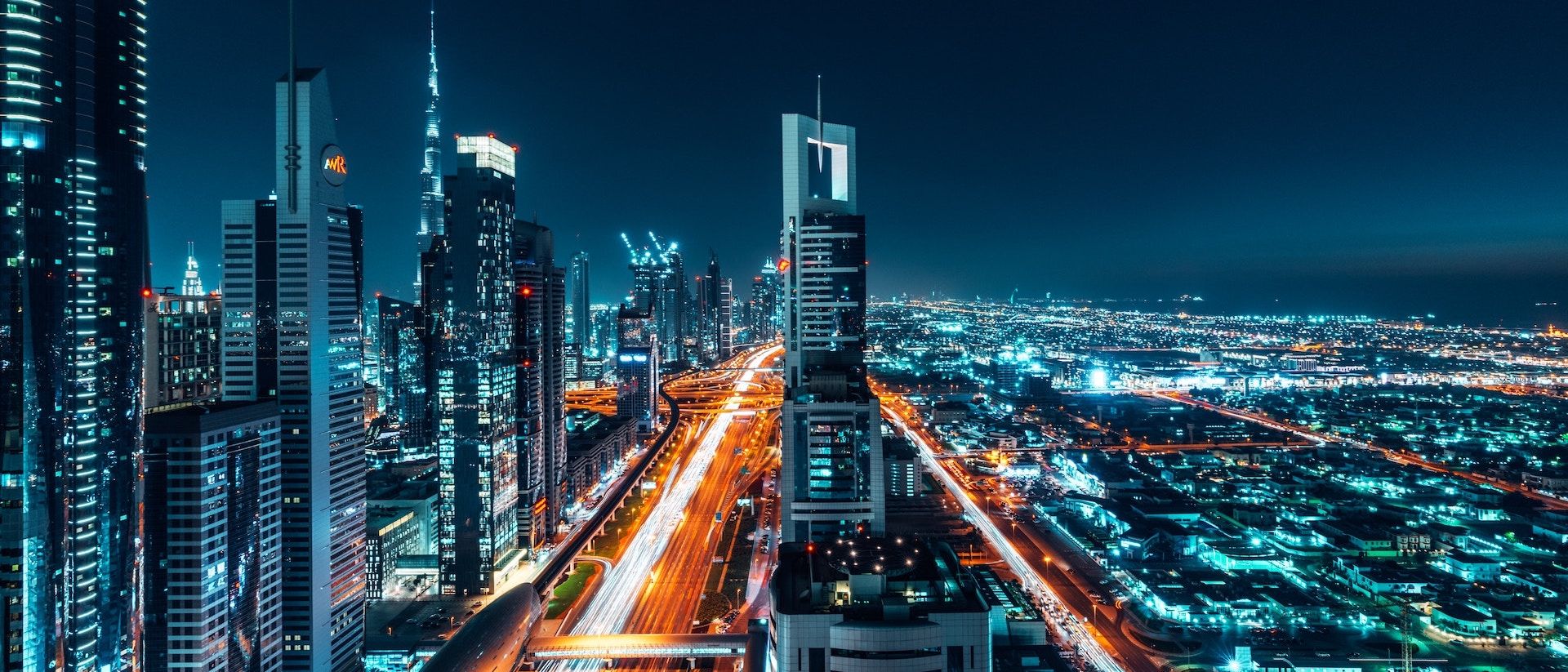
While you could choose to ignore these lessons, it will be more challenging to build relationships, and it might even prevent you from doing your job. Yet no matter the industry, one area is far less affected by these differences, namely data and analytics. While data might come in many shapes – or is that formats? – and sizes, the challenge of analytics is universal. How should we use our data to make better decisions?
Whether you are working for a manufacturer trying to forecast how many components you need to procure, a movie studio planning for the distribution of their latest work, or a retailer preparing to expand their footprint – the fundamental process and ingredients are relatively immutable. I caveat this because data types vary, and labels are often domain specific, but the similarities greatly outweigh the differences.
This is exciting because in many roles, people tend to remain in their industry or market. If you are an expert at maintaining drilling rigs, you are unlikely to suddenly move into a role job tuning track cars. Yet in data, analytics, and AI, you have great freedom. If you want to experience another industry, you have the opportunity to do so, provided you are comfortable working with new data sources, and maybe models.
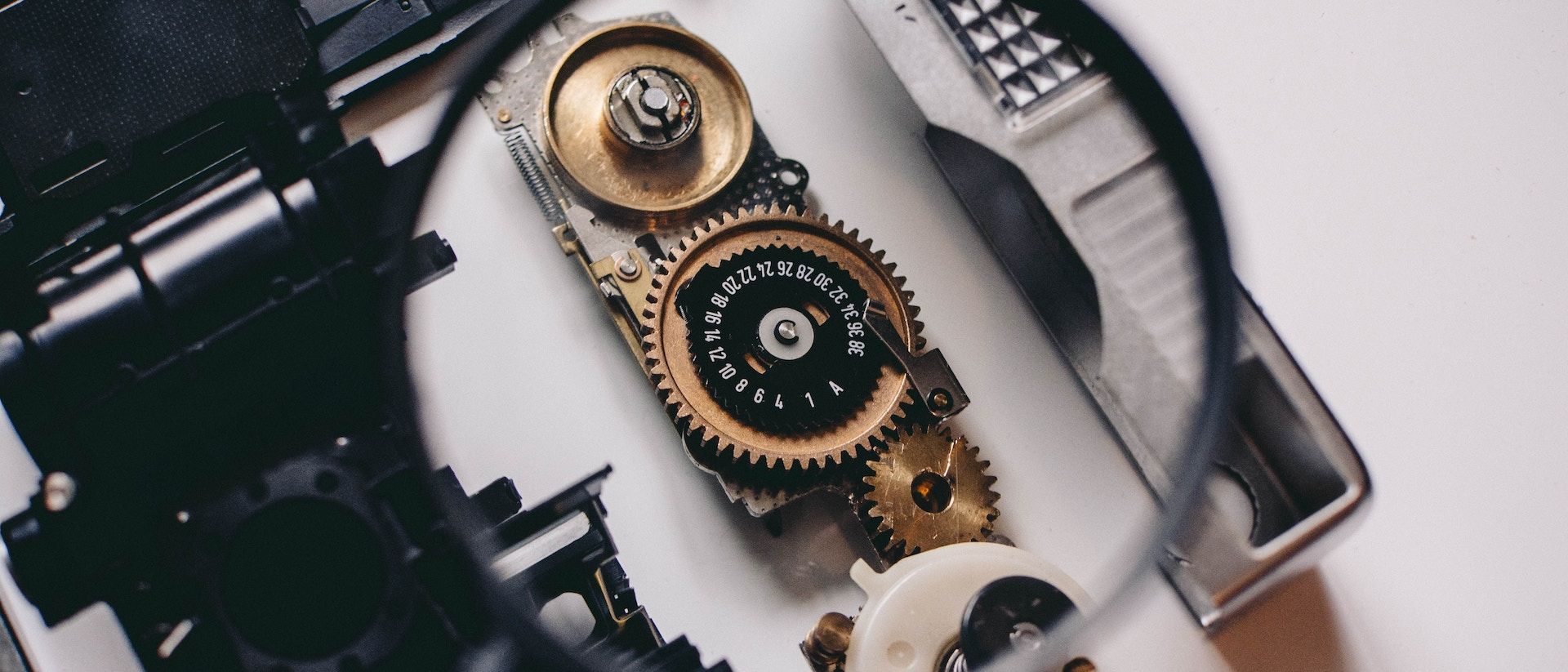
This universality also means that it becomes much easier to learn from others. Best in class analytics frameworks, technology platforms, and skills curricula can be adapted to any industry, subject to regulatory restrictions. This greatly accelerates the development of analytics capabilities. It helps that the analytics and AI community is generally a friendly one, with people eager to share what they have learned.
Finally, the standardisation of the field means that it becomes easier to promote transparency and explainability of analyses. While nice to have when it comes to performance metrics in organisations, this is critical when it concerns data ethics and AI governance in society. This might be the most important point. After all, what is the use of better decisions if these decisions do not feel fair, or are not trusted by anyone?
– Ryan
Q* - Qstar.ai Newsletter
Join the newsletter to receive the latest updates in your inbox.