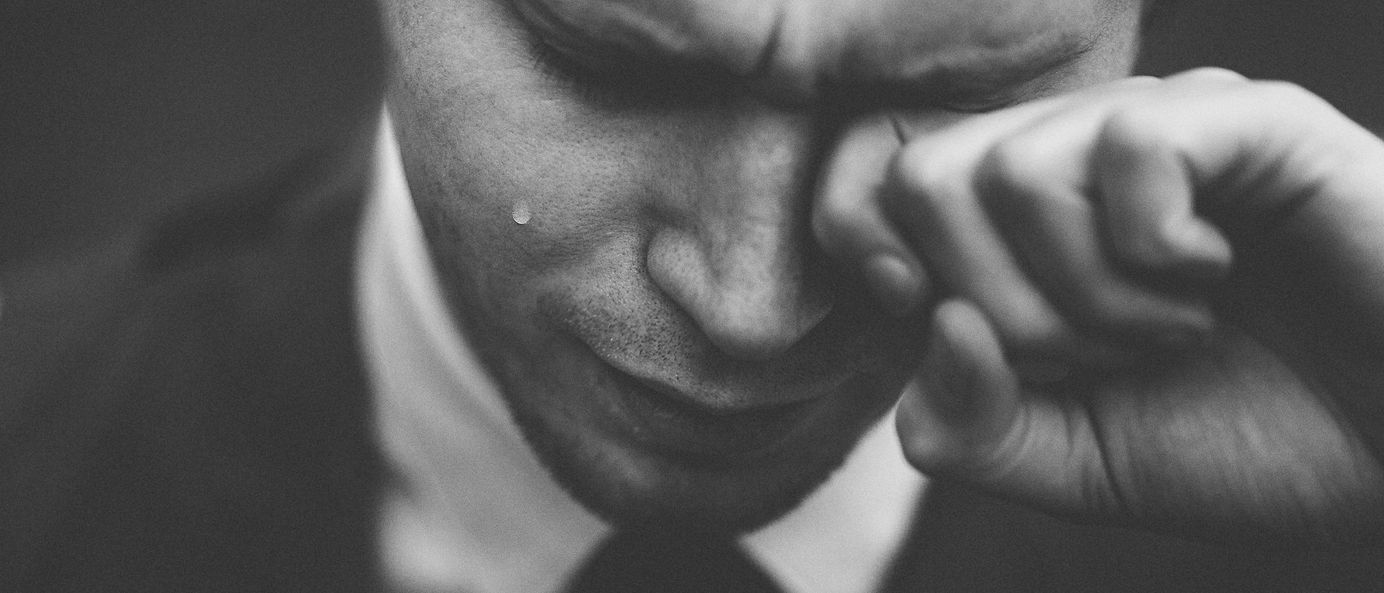
When Not to Use Analytics
In my fifty weekly posts so far this year, almost every single one has advocated for the application of analytics and artificial intelligence (AI). While this is often the most sensible course of action, it is just as important to consider when not to use data. As they say: if all you have is a hammer, everything looks like a nail, and if you think like a statistician, everything looks like a data frame awaiting summary statistics. So when should we exercise analytical restraint?
When the discussion is emotional, not logical. While it is tempting to think we are always rational, our limbic system plays a critical role in our decision-making. Many discussions, both at home and at work, are not truly fact based. In these scenarios, giving others the space to express themselves and making them feel heard is far more important than being factually correct. Instead of focusing on analyses, focus on the human connection.
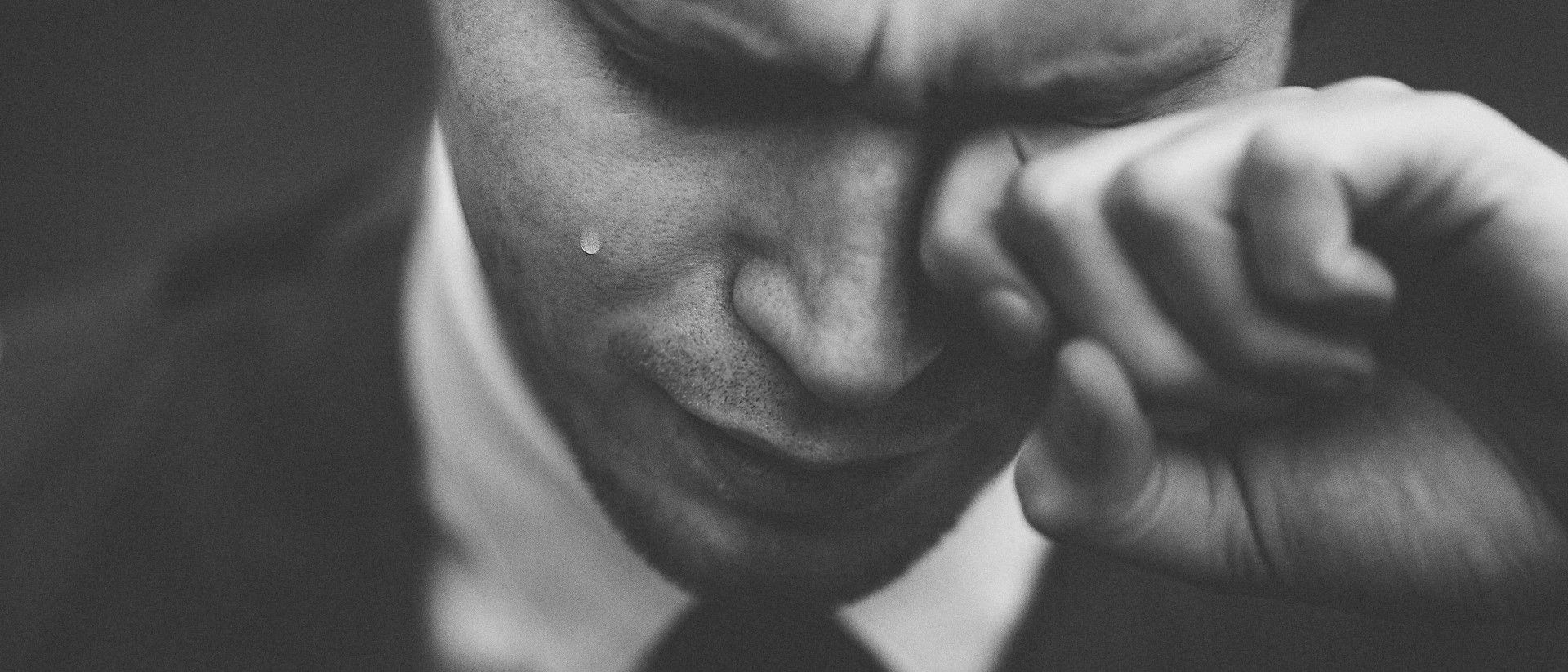
When the process is truly random. I always find it incredible when people present models that are supposedly able to predict complete random events. One website I found shares techniques to analyse "hot", i.e. winning, numbers in roulette. They mention that "statistically speaking, if players are able to [determine the pattern], they can derive very valuable information." And, if I was able to determine the winning lottery ticket, I would own a yacht.
When the problem is not well defined. There is a certain hunger data scientists feel when confronted with a new question, data set, or domain. The urge to jump straight into analyses of the data is sometimes irresistible. Yet it is important to consider whether the problem to be solved has been adequately defined. Do you know what success looks like? Unless this is truly just a learning exercise, it might be better to keep your powder dry.
When the data you have is not representative. This is worth calling out, given the increasing popularity of AI in consumer contexts. While you might have enough data at your disposal to train a solid predictive model, this model could be horribly biased against certain segments of the population if you do not have representative data. In these scenarios, it can be better to retire your model entirely, lest stakeholders misuse or misinterpret it.
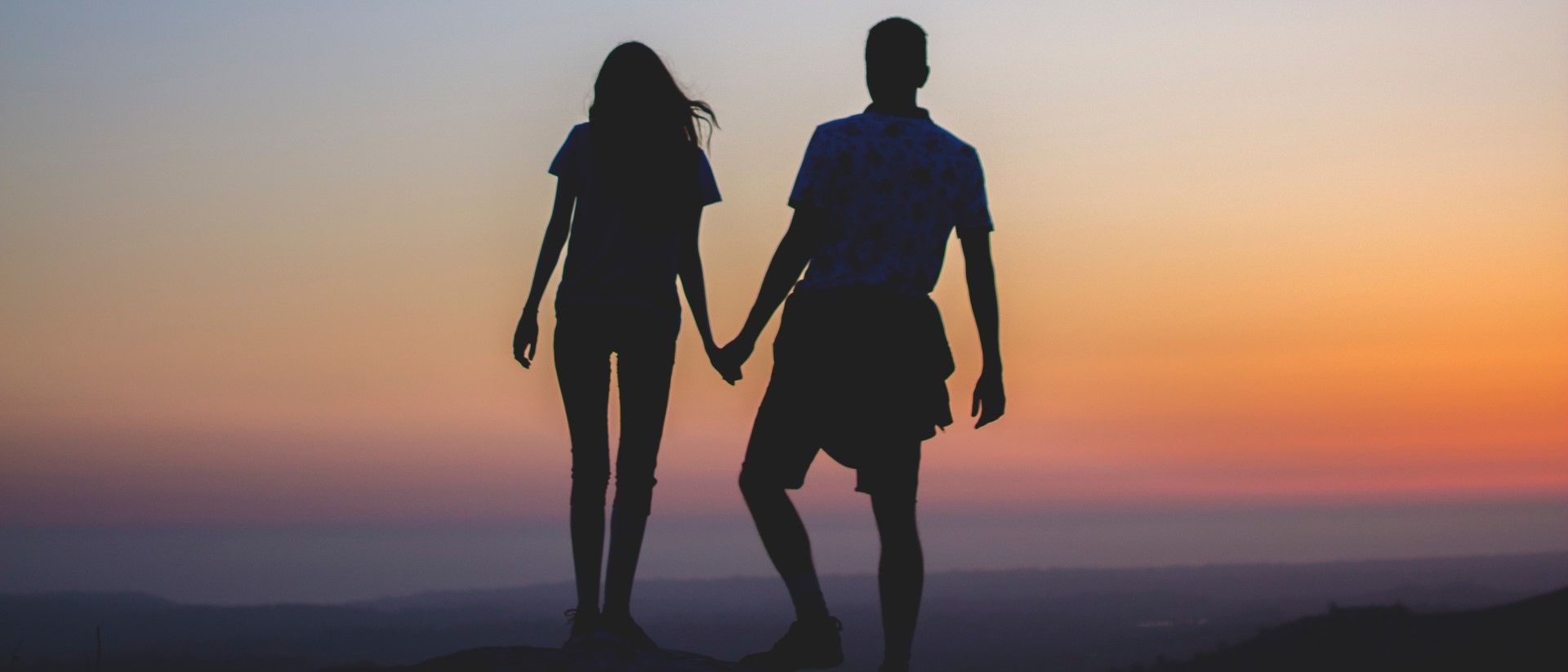
Where stakeholders do not want to think critically. In organisations where people feel the pressure to jump on the analytics and AI bandwagon, it is important they invest the time to interpret and critically assess any outputs. Ensuring the quality of machine learning models takes time, as does the interpretation of statistical findings. Otherwise, stakeholders might just end up advocating for a reduction in US science and technology spending! (see link)
If you are an analyst or a data scientist, rejoice! You find yourself surrounded by exciting opportunities to apply your skills. Yet whether you will be successful in your career will likely also be determined by when you choose to not use data. Paying heed to these signals will not only save you time and effort, but also prevent you from giving the organisation a false sense of confidence through faulty analyses. After all, quality of output always trumps quantity of output.
– Ryan
This week's topic was suggested by Soren Riber. Thank you!
Q* - Qstar.ai Newsletter
Join the newsletter to receive the latest updates in your inbox.