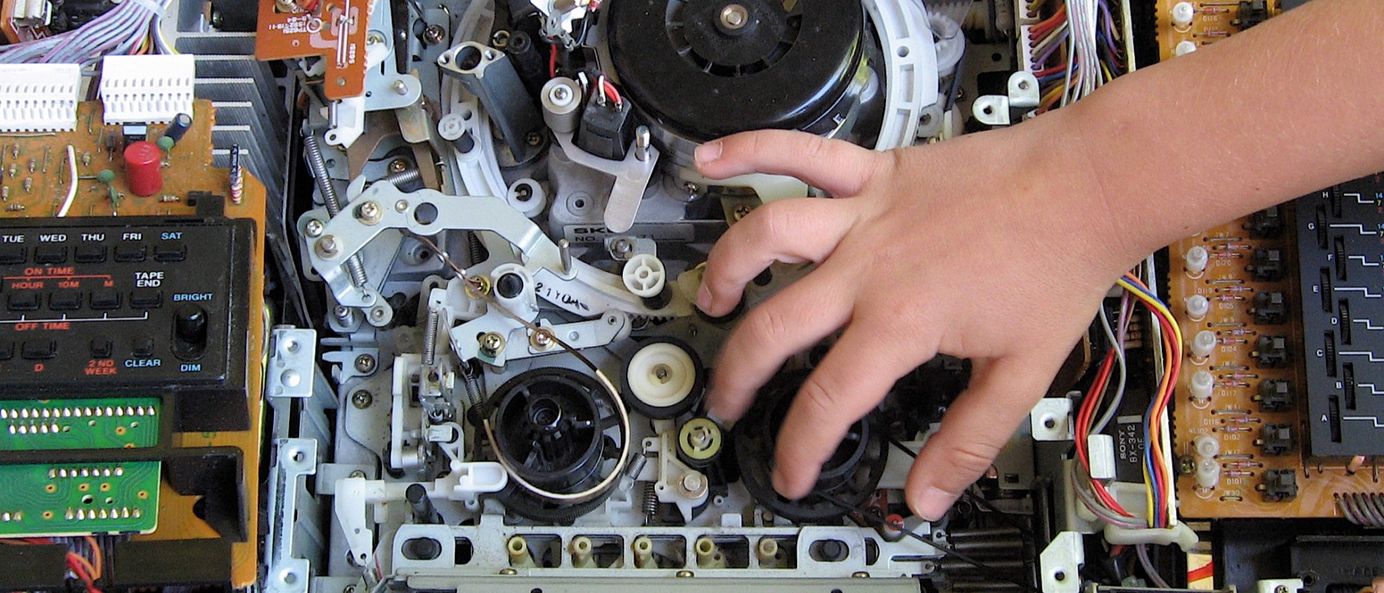
Yes, Machine Learning is Cool. But is Your Organisation Ready Yet?
FOMO, or fear of missing out, is a powerful phenomenon. It is like a type of hunger that gnaws at your soul, a constant pressure at the back of your mind, letting you know that no matter what it is you are doing at this moment — you are not a part of it. For many organisations, the it at this moment in time is artificial intelligence, or more precisely, machine learning. AI FOMO is rife.
It is hard to argue with the renaissance we are seeing in the AI field thanks to breakthroughs in deep learning and distributed computing, but the question is whether this FOMO is justified. Are organisations rightly making significant investments spinning up machine learning efforts in order to “be a part of it?” Do they understand why they are doing this? Are they following a strategy?
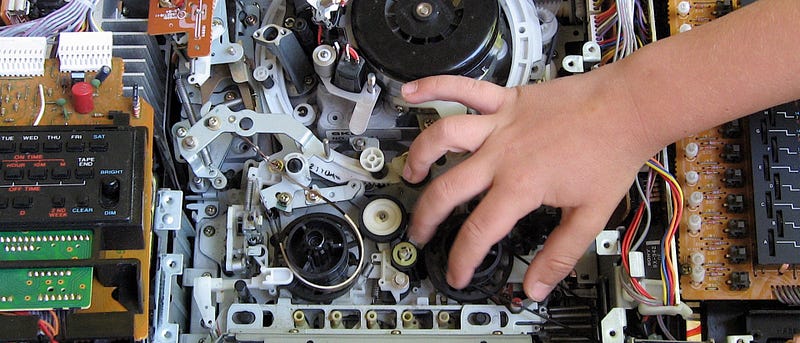
The challenge in part is that, while it is increasingly easy to get started with machine learning, thanks to frameworks such as Tensorflow and PyTorch, this does not mean that organisations always have the data, skills, and capabilities to leverage these properly. Without these critical ingredients, the chances of organisations seeing a return on their machine learning investments is slim.
After all, despite frequent discussions of technology’s ability to catalyse and to disrupt, technology is just technology. What matters, and what decides the value created, is how this technology is utilised. In few areas is this more apparent than in the field of AI. Not only does a machine learning model mean nothing until it is deployed, but it also has to be trained and tuned first.
The primary challenge here is that most organisations do not have neatly labelled data sets lying around, waiting to be fed into a model. In many scenarios, you can count yourself lucky if there is someone in the business who can explain what certain fields in a database mean. If you are trying to build a model to discriminate between a large number of classes this problem is even worse, given the increased size of the training data set required.
Secondly, training and tuning models is difficult, even for those who have significant experience in this area. Many analysts who are tasked with “using machine learning” in organisations do not understand the difference between training, validation, and test data. Most will never have heard of k-fold cross validation. Overfitting becomes a common occurrence when learning curves, regularisation, dropout layers, and data augmentation are foreign concepts.
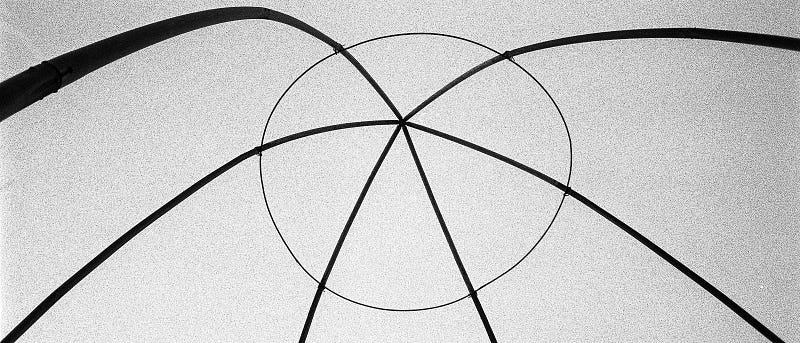
Finally, even if the right level of expertise exists in terms of model building, deploying and managing models in production is another thing entirely. Particularly when it comes to consumer facing applications, it is critical to not only manage performance, but to monitor model drift and periodically retrain the model with new data. This requires infrastructure, processes, and skills.
Organisations should rightly feel inspired and excited by the vast potential of machine learning and artificial intelligence techniques. However, they should understand that it takes both time and focused investment to harness these effectively. In the meantime, thinking about the right questions, ensuring that their data is of good quality, and giving linear regressions another look will likely lead to better returns on investment than rash machine learning efforts.
— Ryan
Q* - Qstar.ai Newsletter
Join the newsletter to receive the latest updates in your inbox.