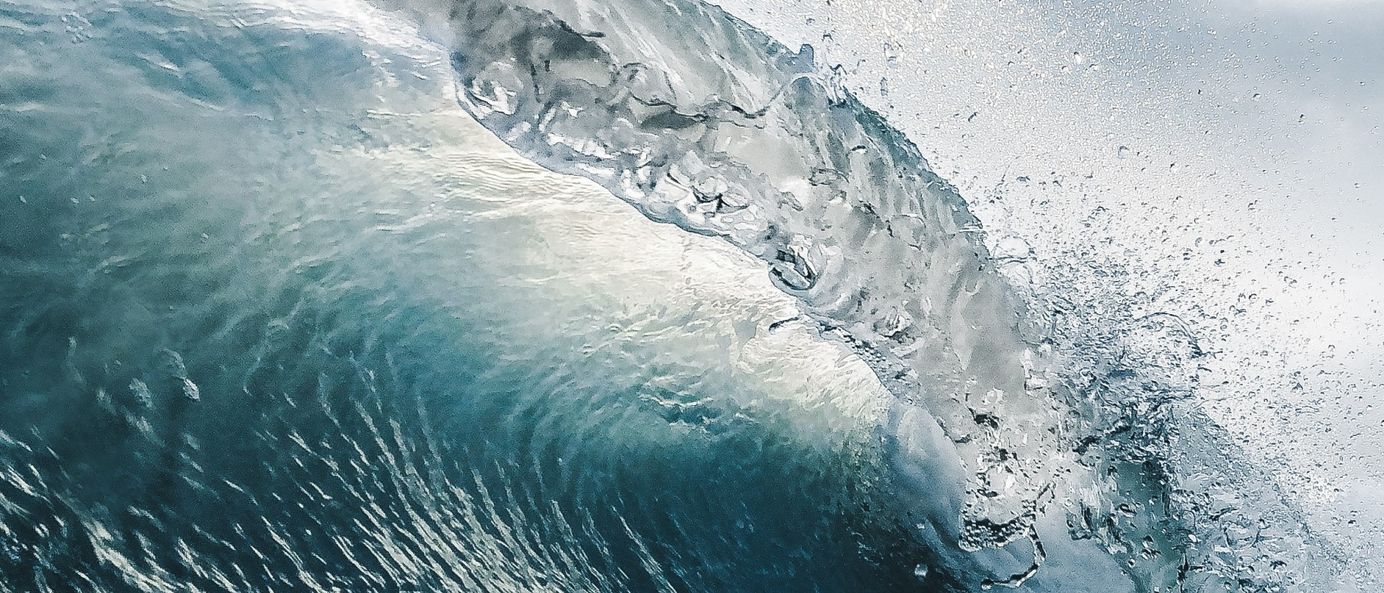
Care About Data Quality? Then Prepare to Let It Go
Ah, data quality: almost as popular a topic as tax returns. While people will gladly converse about the weather at company events, try to bring up data quality more than once and you can find yourself struck off the guest list. Yet data quality is critical. Organisations need to avoid empty data calories, invest in data quality for AI, or pay the price. Let us look at what this means in practice. How can data leaders help make enterprise data quality a success?
To start, let us consider what we mean by data quality. In my opinion, high quality data is data that is fit for purpose, understood, and reliable. While technical measures such as completeness, freshness, and representativity exist, it is difficult to define high quality data based on these alone. For example, while some machine learning algorithms require a high degree of completeness (e.g. LSTMs) other algorithms (e.g. SVMs) can effectively handle sparse data.
Data is fit for purpose when its technical measures are up to the standard required by the use case, e.g. forecasting. For proper management of data, its definition and provenance need to be understood. Finally, the processes and platforms involved in collecting, preparing, and serving this data need to be reliable in order to guarantee the ongoing understanding and fitness of the data. Without these factors it will be difficult for the business to trust the data.
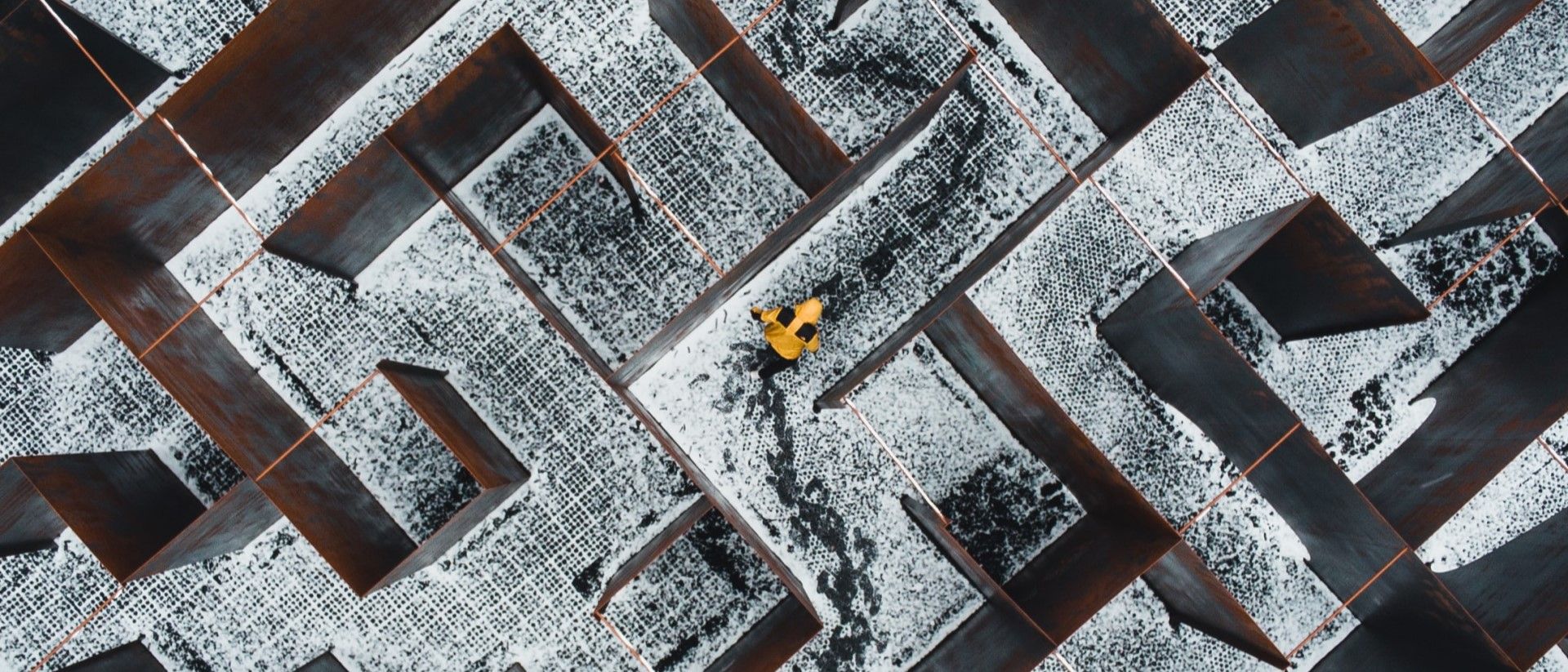
So what role should you as a data leader be playing – or not – in all this?
If you are a Chief Data Officer, you have likely taken charge of many important initiatives: from introducing machine learning, to nurturing product culture, leading cloud migrations, recruiting rock star talent, and architecting critical datasets. However, while a data team can own most of these activities, the same cannot be said for data quality. From data definitions to data capture, accountability for these needs to ultimately sit with the business.
Therefore, it is key to appoint data owners, senior business leaders accountable for data within a domain, e.g. the CFO for finance data. They are assisted by data stewards, who coordinate data quality initiatives (e.g. scorecarding) on behalf of the data owners. These important business roles can be supported by data quality analysts, who diagnose root causes of data quality issues. These analysts can either sit in the business or in the data team.
Business ownership should not preclude data leaders from championing the need for data quality initiatives and their associated resource requirements. However, whether in strategy sessions or budget planning, be prepared to let the topic go if the business is not fully bought in. While I will fight tooth and nail for data architects or security engineers, I am happy to drop data quality roles from the budget if I sense a lack of buy-in/interest from the business.
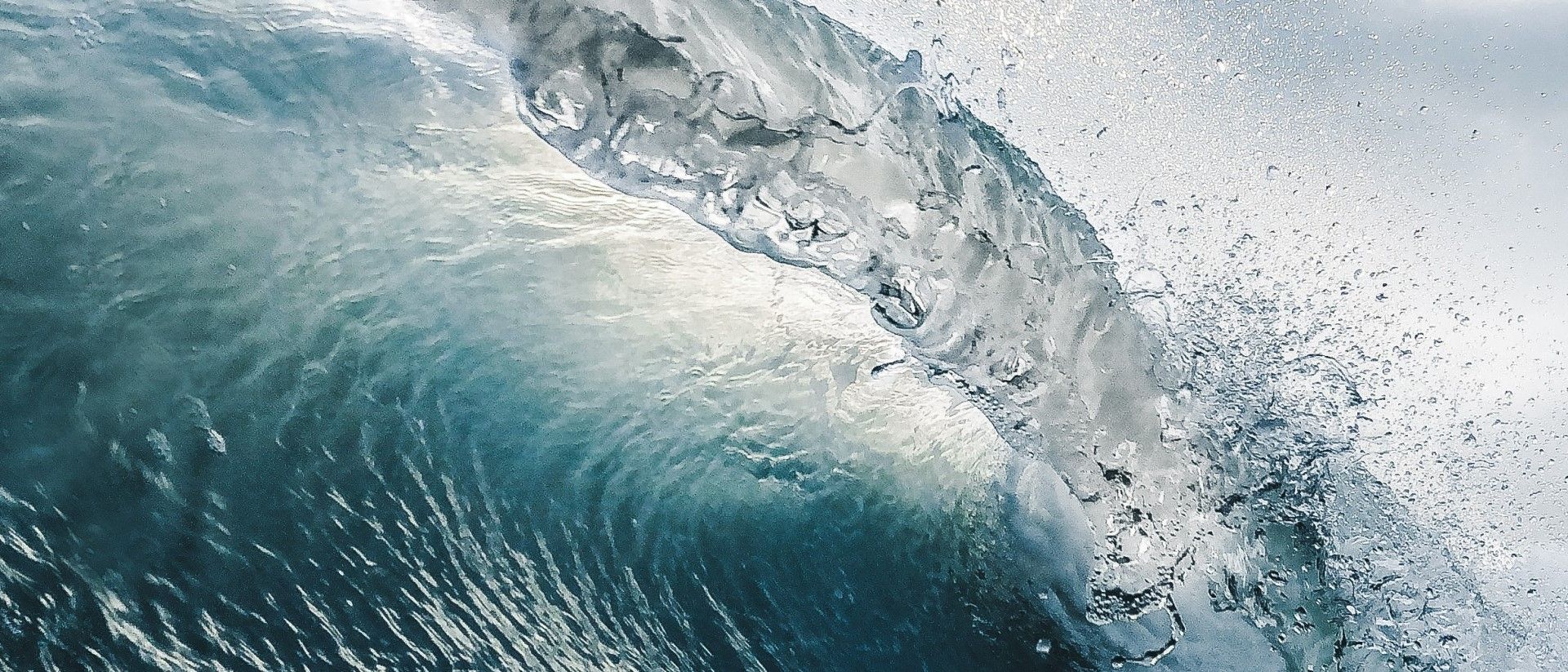
Refusing to take ownership of data quality issues might seem a passive aggressive strategy, but there is no alternative. A data team can build the best platform, pipelines, and products, but they cannot magically solve data quality issues. A simple example is that of a single customer view – you can have a great data model but if employees do not care about collecting valid email addresses or phone numbers from customers, why even go on this journey?
Some salespeople will hold forth on how their software will solve your data quality problems. While I am not in the business of vendor ratings, suffice to say that while good software can help improve data quality, it can only ever be a catalyst, not a fix. Fundamentally, data quality problems require people to take accountability for the data they collect, manage, and consume. If people choose to ignore this fact, well, you know what they say about poor craftsmen.
In our world of increasing algorithmic complexity, data quality is more important than ever. We need to therefore be honest with ourselves and our organisations. Pretending clever engineering will solve structural data quality issues is arguably as harmful as pretending these issues do not exist in the first place. While it might be painful, if you as a data leader truly care about addressing data quality issues, it might be time to stop holding on to them.
– Ryan
Q* - Qstar.ai Newsletter
Join the newsletter to receive the latest updates in your inbox.